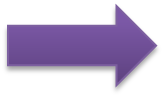
Documentation
- Overview
- EpilepsyGene is a comprehensive genetic database for genes and variants related to epilepsy. It contains such genetic factors as SNVs, InDels and CNVs. Data in this database were derived from both profound literature screenings and extended functional analyses, such as ANNOVAR annotation, Gene Ontology (GO), protein interaction network (PPI) and Pathway analysis. A full annotation of each genetic factor was made, and the correlations among these genetic factors were demonstrated to make EpilepsyGene a comprehensive and connected information resource for further genetic studies of epilepsy. EpilepsyGene provide several ways to search (part 4) for the thought of facilitating users to access data they want. Additionally, browse (part 5) was also incorporated to facilitate browsing.
- Data collection
- We performed a comprehensive search in PubMed for studies related with epilepsy, and a total of 818 publications were collected. Details of variants, like phenotype, gene symbol, cDNA change, ethnicity, gender and age at onset, were extracted from original publications. Additionally, relavent variants in MITOMAP, the Lafora database, MeGene and epiGAD were also integrated into EpilepsyGene.
- Data analysis
- Annovar annotation
- Based on data collected from publications, we performed ANNOVAR analysis, which can generate detailed information of variant, like gene annotation, amino acid change annotation, SIFT scores, PolyPhen scores, LRT scores, MutationTaster scores, PhyloP conservation scores, GERP++ conservation scores, dbSNP identifiers, 1000 Genomes Project allele frequencies, NHLBI-ESP 5400 exome project allele frequencies and other information. Users can get annotations above by clicking hyperlinks in the column 'EG ID'. Program used to convert coordinate from CDS to genome can be accessed at here.
- Gene prioritization
- Based on the annotations made by ten functional prediction tools (i.e. SIFT, phyloP, SiPhy, LRT, MutationTaster, MutationAssessor, FATHMM, GERP++ PolyPhen2_HDIV and PolyPhen2_HVAR), gene prioritization was conducted to identify high confidence genes by the relevance to epilepsy. Score 1 was assigned to the variant whose prediction is 'Deleterious', 'Disease causing', 'Tolerated' or 'Conserved', and 10 was assigned to the variant whose mutation type (MT) is 'frame shift', 'splicing', 'stop gain' or 'stop loss'. The score of the variant is then calculated by:
- where x is the number of software which predict the variant as 'Deleterious', 'Disease-causing', 'Tolerated' or 'Conserved'. The final score of the gene is the sum of the score of all variants in the gene:
- where N denotes the number of mutations in the gene, and Si represents the score of the variant. Ultimately, 154 high confidence genes were obtained on the condition that the total score of the gene (Sg) was no less than ten.
- Functional analysis
- Based on the prioritized genes, a variety of functional analyses were performed, including co-expression analysis, phenotype enrichment, GO enrichment, pathway enrichment and PPI enrichment.
- Co-expression analysis
- To explore the expression pattern of 154 genes, WGCNA was used to conduct co-expression analysis by adopting brain expression levels from RNAseq. The genes were then classified into four gene sets, each presenting a different expression pattern in different periods and areas of the brain.
- Figure 1
- Phenotype enrichment
- To identify epileptic phenotypes associated with the four gene sets, the enrichment of phenotypes was performed using the hypergeometric test (described in WebGestalt), with significance threshold of p.0.01 and minimum number of genes for a phenotype being three.
- Figure 2
- Gene Ontology
- Gene Ontology annotation, including such three parts as Cellular Component, Molecular Function and Biological Process, was performed by WebGestalt 2.0. Each part contains the GO term accession, GO term name, Ratio of enrichment, P-Value , Adjusted P-value and Genes related. Hyperlinks were provided in column 'Genes related' ( Figure 1 ), which will show detailed information in EpilepsyGene about that gene.

- Figure 3
- Pathway analysis
- Enriched pathway information was accessed through WebGestalt 2.0, which includes KEGG analysis, Wikipathways analysis and Pathway commons analysis. Each part contains Term, Ratio of enrichment, P-value, Adjusted P-value and Genes related.

- Figure 4
- Protein interaction network
- We peformed protein interaction network module analysis by WebGestalt 2.0. Figure 3 shows the most statistically significant PPI. The circle in green represents epileptic genes involved in this PPI.
- Figure 5
- Overlap analysis
- For the purpose of exploring the comorbidity of other disorders (autism spectrum disorder-ASD, attention deficit hyperactivity disorder-ADHD, mental retardation-MR, schizophrenia-SCZ) with epilepsy, overlap analysis was carried out based on the shared genes (Figure 6), intersectional epileptic phenotypes (Figure 7) and enriched pathways (Figure 8).
- Shared genes
- Genes in three existing databases (AutismKB, ADHDgene and SZGR) were firstly collected and then compared with genes in EpilepsyGene. Since there are no genetic resources specific for MR, HGMD was chosen as the database to collect MR-related genes.
- Figure 6
- Intersectional phenotypes
- To identify the specific phenotypes associated with the genes overlapped by epilepsy and ASD/SCZ/ADHD/MR, epileptic phenotypes associated with the common genes were retrieved and classified.
- Figure 7
- Enriched pathways
- To investigate the biological implications indicated by the overlapped genes, pathway enrichment analysis for the common genes was undertaken separately by WebGestalt, figure 8 shows the the top three enriched pathways in each data set.
- Figure 8
- Mutation spectrum
- To graphically display mutations in genes, SVG was used to achieve the visualization of mutation spectrum. Figure 4 shows a demo mutation spectrum. The word in red represents the variant is reported mone than once or associated with more than one phenotype.

- Figure 9
- Gene-disease network
- Gene-gene associations
- To graphically display associations between two epileptic genes, SVG was used to achieve the visualization of gene-gene network. Figure 10 shows a demo gene-gene network.

- Figure 10
- Disease-disease associations
- To facilitate the exploration of the exceptional genetic heterogeneity of inherited retinal diseases, gene-disease network, implemented by SVG, was completed to graphically and vividly show intrinsic relations between epileptic genes and epilepsy. Figure 11 shows a demo gene-disease network.

- Figure 11
- Gene-disease associations
- To facilitate the exploration of the exceptional genetic heterogeneity of inherited retinal diseases, gene-disease network, implemented by SVG, was completed to graphically and vividly show intrinsic relations between epileptic genes and epilepsy. Figure 12 shows a demo gene-disease network.

- Figure 12
- Search
- Advanced search
- Advanced search contains four parts: (i) 'Variant search'; (ii) 'Disease search'; (iii) 'Literature search' and (iv) 'Batch search'. SNVs, InDels and CNVs can be easily accessed through 'Variant search'. 'Disease search' is used to get phenotype of interest by keywords search. 'Literature search' is designed to obtain articles of interest, like articles on mutations detected by whole-exome sequencing. Last but not least, 'Batch search' will be useful if you want to fetch genetic variants of more than one genes with multiple conditions to filter out unwanted data.
- Figure 13
- Quick search
- Figure 14
- Blast search
- Figure 15
- Browse
- To facilitate users to browse the data in EpilepsyGene, three different approaches are provided: (i) browse by gene; (ii) browse by mutation; (iii) browse by phenotype and (iv) browse by chromosome. The 'browse by gene' contains all epileptic genes and shared genes with other neurodevelopmental disease, like autism, mental retardation, ADHD and schziprenia. The 'browse by mutation' includes de novo mutations, inherited mutations, copy number variations and mitochondrial mutations. The 'browse by phenotype' classified some epilepsies into several classes, epileptic genes and mutations belong to a class can be easily accessed. Additionally, users can browse EpilepsyGene by chromosome in a graphical way, in which all the variants are mapped on the chromosomes and linked to gene information page. Figure 16 shows a demo browse.
- Figure 16
Copyright© 2014, EpilepsyGene Team | All rights researved
Last updated: Jul 6, 2014